수학과 커뮤니티
아주대학교 수학과의 새로운 소식입니다.- 공지사항
-
2024.1115
수학과 진단시스템 운영 보조 연구원 모집(25년 1월 - 25년 2월)
- 모집인원: 1명 - 급여: 월 30만원 - 업무: 수학과 진단시스템 운영 보조 - 기간: 2025년 1월 -2025년 2월 - 지원방법: 아래 구글 폼 작성하여 제출 - 지원자격: 생각하는 것을 좋아하고 배우고자 하는 적극적인 자세를 갖춘 아주대 수학과 재학 중인 학부생 - 지원서 제출기한: 11월 30일 17시까지 * 아주산업수학통계센터인턴으로 등록된 경우, 가산점 부여 지원서 링크 https://forms.gle/RxRPH98pmowb5WKC7 문의: 박보람<borampark@ajou.ac.kr>
-
2024.1029
[일정 변경 공지] 2024년 아주대학교 산업수학·통계센터(ACMSI) 데이터 경진대회 안내
-
2024.1007
2025학년도 대학원의 날 참여 안내
▶▶ 설명회 참여 신청 폼 작성하기 (~10/16) 대학원 진학에 관심 있는 학생들의 많은 참여 신청 부탁 드립니다!
-
2024.1115
- 수학과 소식
-
2024.1007
수학과, 규슈대학교 수학과 및 산업수학연구소와 업무 협약 체결
우리 수학과는 일본 규슈대학교 수학과 및 산업수학연구소(Institute of Mathematics for Industry, IMI)와 양 기관 간의 과학, 학문, 교육 협력을 촉진하기 위한 업무 협약을 체결했다. 이번 협약은 상호 간의 관계를 강화하고 공동 연구 및 교육 계획을 위한 새로운 길을 여는 것을 목표로 하고 있다. 지난 9월 28일 라마다프라자 수원 오키드홀에서 열린 협약식에서 박보람 수학과 학과장과 규슈대학교 산업수학연구소 Fumio Hiroshima 연구소장이 협약서에 서명하고 이를 교환했다. 이번 협약을 통해 양 기관은 ▲학술 및 행정 직원과 학생 교환 ▲ 공동 연구 및 교육 프로그램 개발·운영 ▲ 학술 정보 및 간행물 교환 ▲ 양 기관이 합의한 기타 학술 교류 등을 협력하여 진행할 예정이다. 한편 협약식에 앞서 '2024 Ajou-Kyushu-NIMS Joint Workshop on Industrial and Applied Mathematics‘이 개최됐다. 지난 9월 27일부터 3일간 진행된 이번 행사에는 ‘2024 아주-규슈 응용수학 여름학교(2024 Ajou-Kyushu Summer School on Applied Mathematics)’의 성과 발표와 더불어 교수, 연구자, 학생 60여명이 모여 응용수학과 산업수학 분야의 아이디어와 네트워크를 공유했다. 이번 행사는 박형주 교수의 공개 강연으로 시작되었으며, 초청강연 6회, 구두발표 7회, 포스터발표 15회 등 다양한 학술세션으로 진행됐다.
-
2024.0926
[안내] 2024 Ajou-Kyushu-NIMS Joint Workshop on Industrial and Applied Mathematics
국내외 산업 및 응용수학 분야에서 학문과 교육 연계를 강화하기 위한 워크숍을 개최합니다. 아주대학교와 규슈대학교는 2018년부터 학술 교류를 이어왔으며, 지난 여름에는 규슈대학교에서 「2024 Summer School on Applied Mathematics」을 공동으로 개최했습니다. 이번 워크숍에서는 여름 학교의 교육 성과를 공유하며, 산업 및 응용수학 분야의 네트워크 형성을 도모하고자 합니다. 많은 관심과 참여 부탁 드립니다. 행사명 : 2024 Ajou-Kyushu-NIMS Joint Workshop on Industrial and Applied Mathematics - 일시 : 2024년 9월 27일(금) ~ 29일(일) - 장소 : 아주대학교 팔달관 311호 & 수원 라마다호텔 오키드 홀 [대중강연] - 일시 : 2024년 9월 27일(금) 오후 4시 30분 ~ 5시 30분 - 장소 : 팔달관 311호 - 연사 : 박형주 교수님(아주대학교) - 주제 : Unreasonable effectiveness of pure mathematics - 초록 : Mathematics that used to be considered pure often addresses real world problems in unexpected and surprising ways. The concept of information entropy helps us to distinguish between valuable and worthless information. A nontrivial group called eliptic curve helped us to settle the longstanding Fermat's Last Problem, and also gave us a cryptosystem being used to protect credit card transactions. Algebraic topology successfully explains the phase transition of 2-dimensional materials like graphene and created a new area called topological physics to which 2016 Nobel Prize was awarded. Topology, by observe the shape of big data, also gave us a new way of predicting/diagnosing diabetes and breat cancer. - 문의 : 031-219-3816 or ahyungoo@ajou.ac.kr
-
2024.0923
2024-2학기 수학과 colloquium 안내[9.26 목]
안녕하세요. 수학과입니다. 9/26 (목)에 예정된 수학과 colloquium에 대해 안내 드립니다. ----------------------------------------------------------------------------------------- 일시: 9월 26일(목) 16:30~17:30 장소: 팔달관 621호 연사: 고승찬 교수님(인하대학교 수학) 제목: Mathematical Theory of Neural Network Approximation and its Application to Scientific Machine Learning 초록: In recent years, modern machine learning techniques using deep neural networks have achieved tremendous success in various fields. From a mathematical point of view, deep learning essentially involves approximating a target function, relying on the approximation power of deep neural networks. Therefore, it is important to understand the approximation and generalization properties of neural networks in high dimensions. The primary objective of this talk is to mathematically analyze the approximation of neural networks within the classical numerical analysis framework. We will explore the proper regularity of target functions which is suitable for the neural network approximation, and investigate how these properties are reflected in the approximation and learning complexity of neural networks. Next, I will apply these theories to my recent work on the operator learning method for solving parametric PDEs. I will analyze the intrinsic structure of the proposed method through the theory described above, deriving some useful results both theoretically and practically. Furthermore, I will demonstrate some relevant numerical experiments, confirming that these theory-guided strategies can be utilized to significantly improve the performance of the method. -----------------------------------------------------------------------------------------------------
-
2024.1007
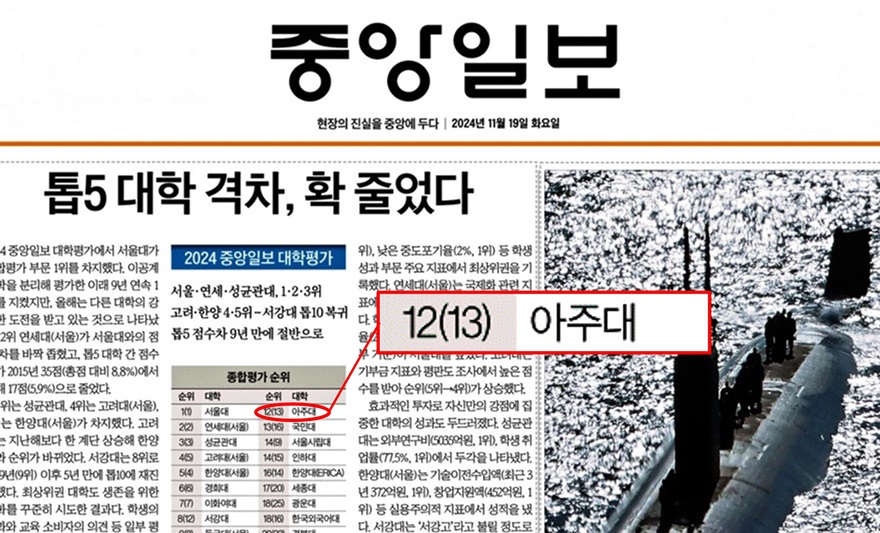
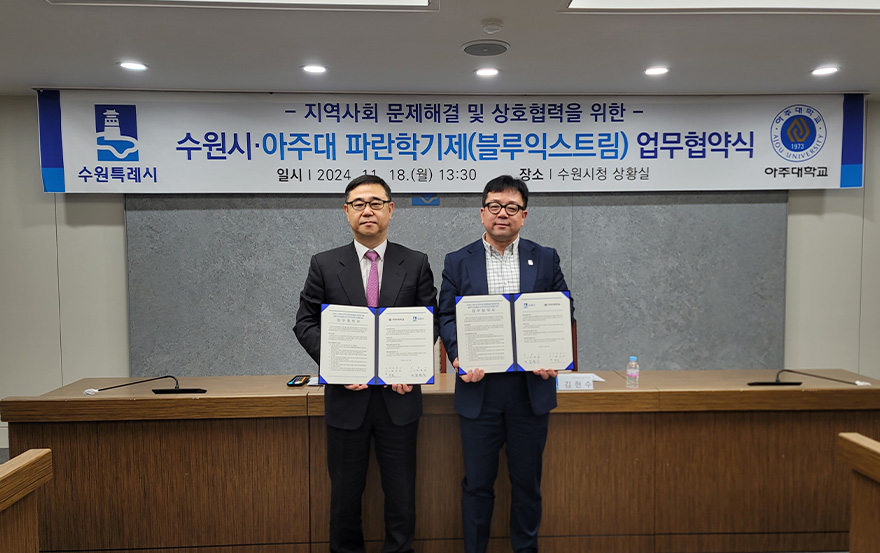
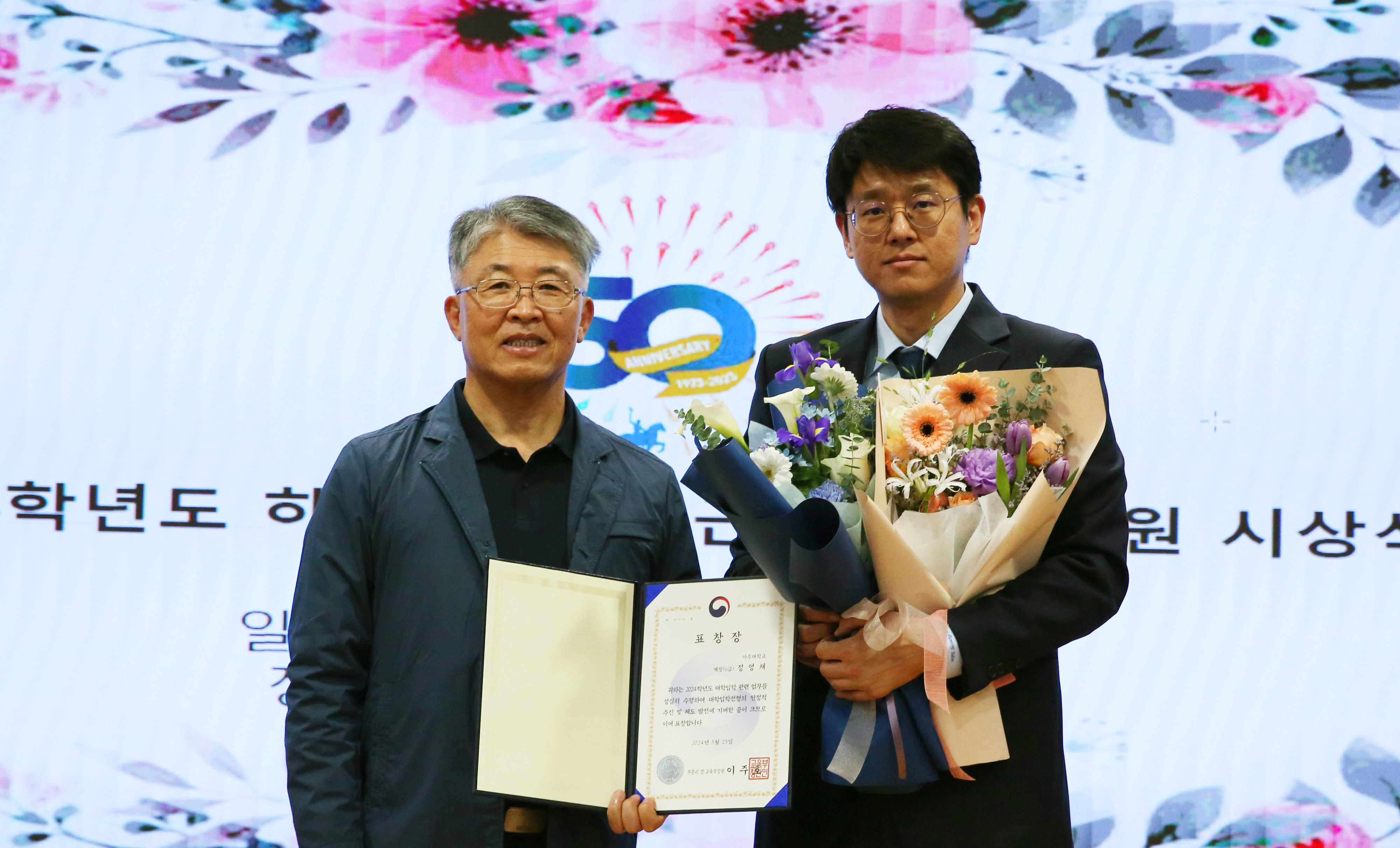
아주뉴스